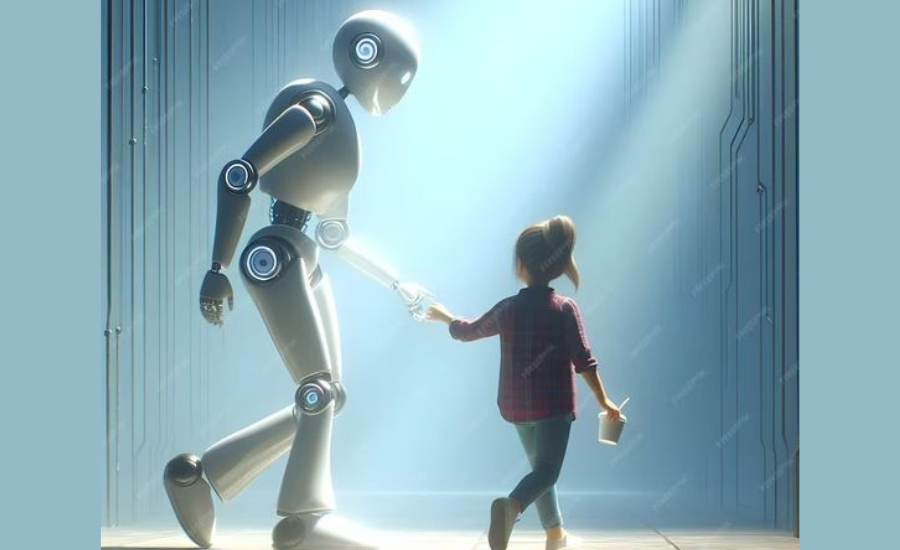
Discover how the Extangiental Quadr empowers researchers and organizations by providing a structured and innovative framework for addressing complex analytical challenges across a multitude of scientific disciplines. This groundbreaking model is designed to facilitate deep analysis by integrating advanced mathematical principles, including quadratic and linear functions, into a cohesive analytical toolkit. As data complexity increases in fields such as healthcare, finance, and environmental science, the Extangiental Quadr equips professionals with the ability to extract meaningful insights from large and multifaceted datasets.
By enabling a multi-dimensional approach to data analysis, the Extangiental Quadr allows researchers to uncover hidden patterns and correlations that traditional analytical methods might miss. For instance, in healthcare research, it can help identify subtle relationships between patient data and treatment outcomes, thereby improving the efficacy of medical interventions. Similarly, in the financial sector, organizations can use the Extangiental Quadr to model market trends with greater accuracy, allowing for more informed investment decisions.
Introduction to the Extangiental Quadr
In an era defined by data, innovative analytical models are revolutionizing industries, enhancing decision-making, and influencing future trends in business and research. Among these groundbreaking frameworks is the Extangiental Quadr. What is the Extangiental Quadr, and how does it integrate into the contemporary landscape of data science?
The Extangiental Quadr serves as an advanced model designed to unveil profound analytical insights from extensive datasets. By harmonizing sophisticated metrics, quadratic functions, and linear models, it emerges as a robust analytical tool for entities aiming to enhance their decision-making capabilities. In today’s competitive environment, the significance of obtaining analytical insights cannot be overlooked, as businesses must adeptly manage and interpret vast data quantities.
Whether your focus is on predictive analytics, financial modeling, or consumer behavior studies, the Extangiental Quadr provides unparalleled advantages, effectively bridging the gap between traditional data processing methods and modern predictive analytics.
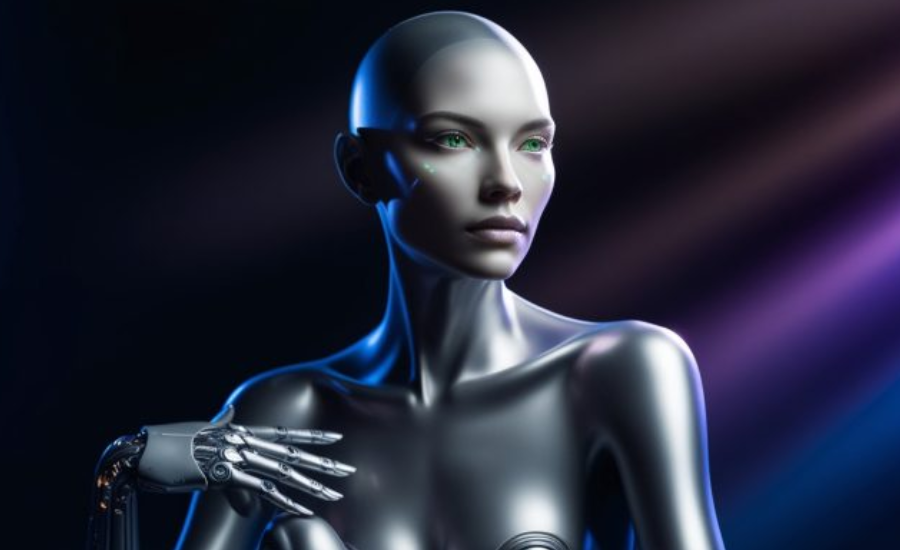
The Evolution of Data Analysis
Data analysis has witnessed a dramatic transformation over recent decades. The progression from basic statistical techniques to advanced analytical frameworks illustrates the demand for deeper insights.
Transitioning from Basic Statistical Tools to Advanced Analytics
Initially, organizations relied heavily on straightforward descriptive statistics for historical data analysis. However, the quest for more actionable insights ignited the development of increasingly intricate models.
The Rise of Complex Analytical Frameworks
With advancements in machine learning and the proliferation of big data, the capacity to analyze complex datasets has surged, leading to the emergence of models like the Extangiental Quadr, which facilitates nuanced understanding and prediction.
Unpacking the Extangiental Quadr Framework
At its foundation, the Extangiental Quadr synthesizes a variety of advanced mathematical principles. Its structured approach enables the simultaneous analysis of data across multiple dimensions, resulting in richer and more comprehensive insights.
Key Components of the Extangiental Quadr
This model effectively integrates quadratic functions, which unveil intricate patterns in data behavior, alongside linear models that simplify complex relationships for better understanding.
Operational Mechanism of the Extangiental Quadr
By leveraging its mathematical elements, the Extangiental Quadr enhances predictive accuracy compared to traditional models, making it invaluable in precision-demanding sectors such as finance and healthcare.
The Mathematical Foundations Behind the Extangiental Quadr
The mathematical underpinnings of the Extangiental Quadr are sophisticated yet immensely powerful, anchored in both quadratic and linear models, enabling versatility across various industries.
Core Mathematical Principles of the Extangiental Quadr
The model employs advanced calculus and linear algebra to navigate complex datasets effectively.
Applications of Quadratic and Linear Models
The interplay of these models facilitates comprehensive predictions, capturing not only linear trends but also intricate non-linear relationships often overlooked by conventional analytical approaches.
Key Metrics for Insightful Analysis
The Extangiental Quadr meticulously analyzes a range of essential metrics to extract meaningful insights.
Primary Analytical Metrics in the Extangiental Quadr
Key metrics include regression coefficients, R-squared values, and error margins, all crucial for assessing the model’s accuracy and reliability.
Impact of Metrics on Strategic Decision-Making
By evaluating these metrics, businesses can refine their strategies, enhance operational efficiency, and make more accurate predictions about future trends.
Real-World Applications of the Extangiental Quadr
The Extangiental Quadr transcends theoretical boundaries, finding practical applications across numerous industries. Its capacity to provide advanced analytical insights revolutionizes decision-making frameworks.
Leveraging the Extangiental Quadr in Business Analytics
Companies can utilize the Extangiental Quadr to anticipate customer behaviors, optimize supply chain logistics, and discern market trends. This model equips organizations with actionable insights derived from intricate data patterns, giving them a competitive edge.
Predictive Modeling in Financial Services
In the realm of finance, the Extangiental Quadr aids in predicting market fluctuations, assessing risks, and forecasting financial performance. Its proficiency in capturing non-linear dynamics is particularly beneficial in volatile market conditions.
Advantages of Implementing the Extangiental Quadr
Organizations keen on enhancing their data analysis capabilities can reap numerous benefits from employing the Extangiental Quadr. These advantages solidify its status as a preferred choice in today’s data-centric landscape.
Improved Data Accuracy with the Extangiental Quadr
The model enables precise data analysis, unveiling patterns that traditional models might miss.
Enhanced Efficiency in Data Processing
By automating segments of the analytical process, the Extangiental Quadr minimizes the time and resources required for processing extensive datasets.
Superior Predictive Capabilities
The combination of quadratic and linear models bolsters the Extangiental Quadr’s ability to accurately forecast future trends, a vital aspect of strategic decision-making.
Navigating Challenges with the Extangiental Quadr
Despite its numerous benefits, utilizing the Extangiental Quadr comes with inherent challenges. Awareness of these issues is crucial for optimizing model usage.
Common Challenges Encountered in Analytical Models
One notable concern is overfitting, wherein the model becomes overly customized to a specific dataset, leading to inaccuracies with new data. Data bias is another significant issue, potentially skewing outcomes based on disproportionate variable effects.
Strategies to Mitigate Overfitting and Bias
To counter these challenges, it’s vital to continually validate and test the model against diverse datasets. Employing techniques like cross-validation can enhance generalizability and ensure reliable results.
Comparative Analysis: The Extangiental Quadr vs. Other Analytical Models
In the realm of data analysis, numerous models exist, yet the Extangiental Quadr distinguishes itself through its unique combination of linear and quadratic components.
Differentiating Quadr-Based Models from Other Analytical Approaches
Unlike basic models that focus solely on linear relationships, the Extangiental Quadr captures both linear and non-linear patterns, providing a distinct advantage in accuracy and analytical depth.
Advantages and Limitations of the Extangiental Quadr
While the Extangiental Quadr excels in its predictive capabilities, it necessitates a higher level of mathematical expertise and computational resources compared to simpler models. Nonetheless, the investment is often justified for sectors where precision is paramount, such as healthcare and finance.
Industries Benefiting from the Extangiental Quadr
A diverse array of industries is harnessing the capabilities of the Extangiental Quadr to leverage advanced data analysis.
Advancements in Healthcare Through the Extangiental Quadr
In healthcare, this model supports predictive diagnosis, enhances treatment planning, and identifies early warning indicators of diseases. By analyzing extensive medical datasets, the Extangiental Quadr significantly improves diagnostic accuracy.
Retail Sector and Consumer Behavior Insights
Retailers deploy the Extangiental Quadr to monitor consumer behaviors, optimize pricing strategies, and forecast product demand. This model provides critical insights into evolving consumer preferences and market dynamics, allowing retailers to maintain a competitive edge.
Integrating the Extangiental Quadr into Your Analytical Processes
To fully exploit the potential of the Extangiental Quadr, careful integration into your data pipeline is essential.
Steps for Successful Implementation
Begin by identifying the datasets to be analyzed, ensuring they are clean and structured. Next, choose appropriate analytical tools and software that accommodate the Extangiental Quadr. Finally, incorporate the model into your data processing framework, ensuring seamless interaction with other tools.
Essential Tools and Software for the Extangiental Quadr
Widely-used data analytics platforms, including R, Python, and MATLAB, offer the necessary mathematical and computational resources for implementing the Extangiental Quadr. A robust data infrastructure is also essential to manage large datasets and execute complex computations effectively.
Success Stories: Case Studies Utilizing the Extangiental Quadr
Numerous case studies illustrate the effectiveness of the Extangiental Quadr across different fields.
Exemplary Business Applications
In a notable instance, a prominent retail corporation leveraged the Extangiental Quadr to forecast customer demand for specific products during peak seasons. Insights from the model enabled the company to streamline its supply chain, preventing excess inventory and stock shortages, ultimately resulting in a 20% sales increase.
Academic Applications of the Extangiental Quadr
In academic research, the Extangiental Quadr has been instrumental in analyzing climate data, revealing complex patterns that traditional models failed to capture. This has facilitated more precise climate forecasting and enhanced understanding of global climate phenomena.
Future Prospects for the Extangiental Quadr in Data Analytics
The future of the Extangiental Quadr appears highly promising, especially as ongoing advancements in artificial intelligence (AI) and machine learning (ML) enhance its analytical capabilities. As organizations increasingly seek to harness the power of data for decision-making, the Extangiental Quadr is set to become an indispensable tool across various sectors.
Forecasts for the Next Decade
As the complexity of data continues to rise, the Extangiental Quadr is projected to play a vital role in innovative fields such as autonomous systems, robotics, and the development of smart cities. Its unique ability to analyze vast datasets with both linear and non-linear relationships enables it to uncover insights that traditional models may overlook.
In the realm of autonomous systems, the Extangiental Quadr will facilitate improved decision-making processes, allowing for real-time responses to changing environments. For example, in autonomous vehicles, the model can help predict and adapt to dynamic traffic patterns, enhancing safety and efficiency.
The Role of the Extangiental Quadr in Smart Cities
The implementation of the Extangiental Quadr in the development of smart cities will further amplify its importance. By analyzing data related to traffic flow, energy consumption, and public safety, the model can assist urban planners in making data-driven decisions that optimize city functions. This approach not only enhances the quality of life for residents but also fosters sustainability by reducing resource consumption.
As the demand for efficient data analytics solutions grows, the Extangiental Quadr is poised to redefine the landscape of data analysis. Its continuous evolution alongside AI and ML technologies will unlock new avenues for innovation, ultimately transforming how industries leverage data for strategic decision-making.
Frequently Asked Questions (FAQs)
Q: What is the Extangiental Quadr?
A: The Extangiental Quadr is an advanced analytical model designed to extract profound insights from complex datasets. By integrating quadratic and linear mathematical principles, it enhances the capabilities of data analysis across various scientific fields.
Q: How does the Extangiental Quadr differ from traditional analytical models?
A: Unlike traditional models that primarily focus on linear relationships, the Extangiental Quadr captures both linear and non-linear patterns, providing a more comprehensive understanding of data and improving predictive accuracy.
Q: In which industries can the Extangiental Quadr be applied?
A: The Extangiental Quadr can be applied across diverse industries, including healthcare, finance, retail, and environmental science, among others. It assists organizations in making data-driven decisions by revealing hidden patterns and correlations within their data.
Q: What are the key advantages of using the Extangiental Quadr?
A: The Extangiental Quadr offers improved data accuracy, enhanced efficiency in data processing, and superior predictive capabilities compared to simpler models. It allows businesses to gain deeper insights into customer behavior, market trends, and operational efficiency.
Q: What challenges should organizations be aware of when implementing the Extangiental Quadr?
A: Organizations may encounter challenges such as overfitting and data bias. It is crucial to continuously validate the model against diverse datasets and employ techniques like cross-validation to enhance the reliability and generalizability of results.
Q: How can organizations integrate the Extangiental Quadr into their analytical processes?
A: Successful integration involves identifying relevant datasets, ensuring they are clean and structured, selecting suitable analytical tools, and embedding the Extangiental Quadr into the existing data processing framework.
Conclusion
The Extangiental Quadr represents a groundbreaking analytical model that enhances data analysis capabilities across various scientific domains by integrating quadratic and linear mathematical principles. This innovative framework enables researchers and organizations to extract meaningful insights from complex datasets, revealing hidden patterns and correlations that traditional methods might miss, especially in sectors such as healthcare, finance, and environmental science. With advantages in predictive accuracy and operational efficiency, the Extangiental Quadr is invaluable for informed decision-making, despite challenges like overfitting and data bias, which can be addressed through continuous validation. As advancements in artificial intelligence and machine learning progress, the Extangiental Quadr is poised to redefine data analytics, particularly in innovative fields such as autonomous systems and smart cities, thus transforming how organizations leverage data for strategic insights and decision-making.
Read Next: cronviter